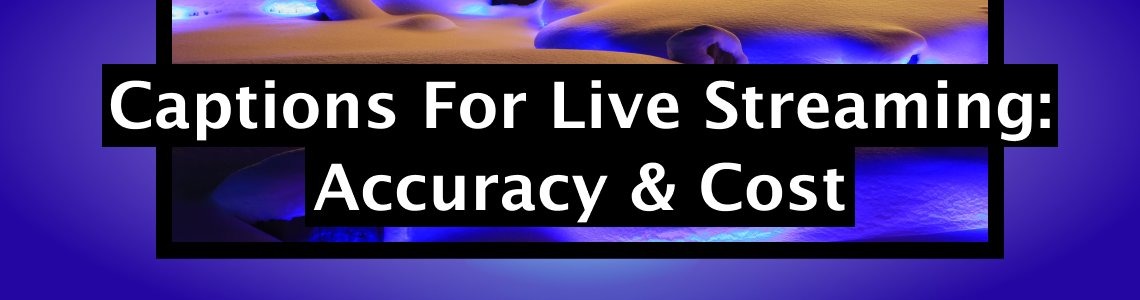
Captions For Live Streaming – Accuracy and Cost
This post is a part of our educational series for those new to Live Captioning.
In this post, we discuss how captions greatly increase the accessibility of your content and examine how accuracy and cost should be considered when selecting a live captioning solution.
Promoting Accessibility
Captions are transcriptions displayed on-screen for viewers who are deaf or hard-of-hearing as well as viewers who aren’t proficient in the language of the live video content. These captions allow viewers to follow what the talent/presenter is saying. Offering captions with live streams has become essential for the following:
- Enterprises building a supportive and inclusive organization.
- Companies in heavily regulated industries such as pharma and medical industries.
- Government organisations (counties, educational institutions) that must comply with legal regulations for equality via accessibility.
Beyond accessibility, viewers in noisy environments or situations where they are unable to play the sound out loud, prefer to watch the live stream muted and read the captions.
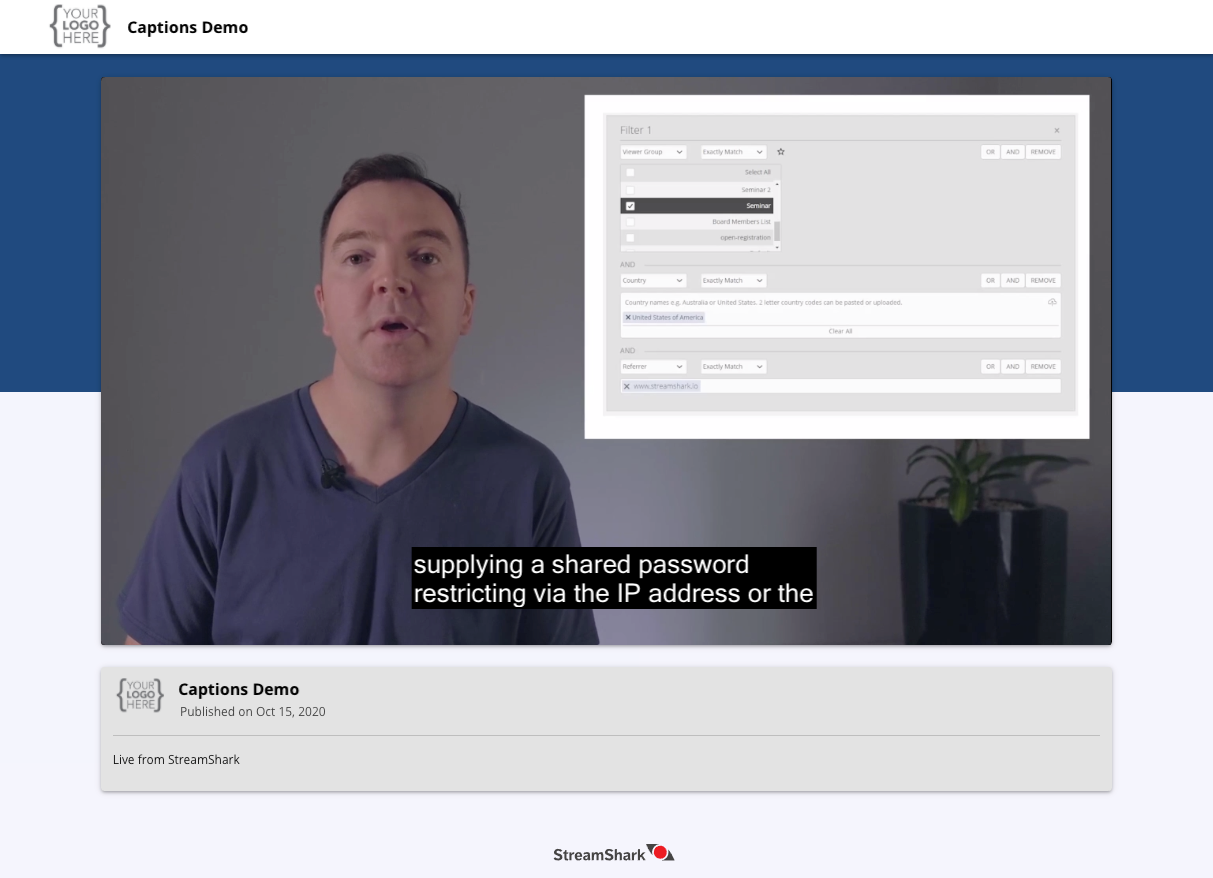
The two most important considerations when evaluating live captioning solutions are accuracy and cost. These are directly impacted by the way the captions are generated, whether the captions are human-generated or computer-generated (leverage Automatic Speech Recognition, commonly known as ASR).
Accuracy of Live Captioning Solutions
In a scenario where live captions are required for a high profile global live stream with VIPs, accuracy is critical and we recommend using human-generated captions. Human captioners excel at understanding human speech (accents, speech variations, technical language, context) and generating the words they hear quickly and accurately.
StreamShark Enterprise customers use professional captioning services such as Ai-Media or EEG Video’s Falcon service. Ai-Media hires and trains their captioners while EEG Video’s Falcon service leverages EEG’s iCap (the global network of caption partners including Ai-Media).
Computer-generated ASR live captions have lower accuracy which may annoy some viewers but are suitable for use-cases such as transcription of everyday meetings where the user can review the transcript and correct it. For solutions leveraging machine learning, the transcription can be assisted with the help of custom dictionaries (containing industry-specific terminology) to train the captioning model. With continued use over a period of time, machine learning based ASR models may achieve an accuracy of 96% to 99%.
EEG Video also offers Lexi, an automatic captioning service with over 90% accuracy in English, Spanish, and French languages. Epiphan Video, one of our Encoder partners, recently released LiveScrypt, a dedicated automatic transcription device leveraging ASR technology with support for English and major European languages.
The models used for measuring accuracy of captions can vary across providers, so it isn’t easy to compare accuracy levels.
Word Error Rate (WER Model)
The most common model used to measure the accuracy of captions has been the Word Error Rate, or WER, model. Word Error Rate (WER) is calculated as follows:
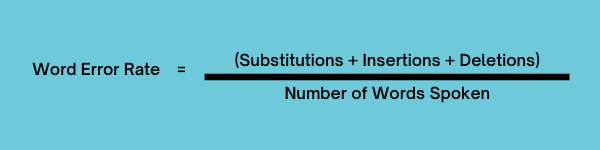
Where,
- Substitutions are any time a word gets replaced (for example, “twist” is transcribed as “wrist”)
- Insertions are anytime a word gets added that wasn’t said (for example, “go-getter” becomes “go get her”)
- Deletions are anytime a word is omitted from the transcript (for example, “let it go” becomes “let go”)
While the WER model is popular, it doesn’t reflect the impact the quality of the captions will have on the viewers, especially those who are deaf or are hard of hearing. While a captioned piece may have high accuracy, it may contain major errors that change the meaning of some of the sentences. It is also possible that there might be a captioned piece with a lower accuracy score but with errors that don’t change the meaning of some of the sentences.
NER Model
The NER model is an alternative to the WER model and was proposed by Prof. Pablo Romero-Fresco and Juan Martinez, a respeaking consultant. ‘Respeaking’ is the term used for a captioner repeating the dialogue of a TV program or other medium into a microphone, which is then turned into captions by text-to-speak software. NER is calculated as follows:
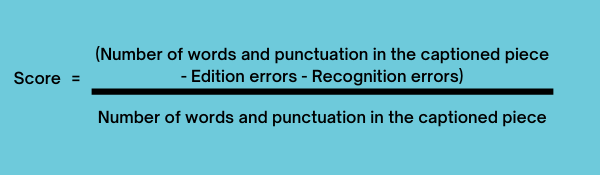
Where,
- Edition errors are words that have been spoken but do not appear in the captions, or words that have been added to the captions but have not been spoken (for example, “let it go” becomes “let go” or “this is chaos” becomes “this is a chaos”)
- Recognition errors are incorrect word(s) appearing in the captions (for example, “twist” is transcribed as “wrist”)
The NER model considers that all errors do not pose the same problems in comprehension. So it considers the impact of the accuracy of captions on the viewers. This measurement process is already used for public television broadcasts in several European countries like Italy and Switzerland as well as in Australia. Ai-Media uses the NER Model and its human-generated captions have been externally audited as having up to 99.6% accuracy.
Cost of Live Captioning Solutions
Different vendors offer different pricing models, including:
- Flat rate charges for booking a human captioner for a half-day/full-day
- Flat rate pricing based on 10 minute slots ($/ten-minutes)
- Flat rate hourly pricing ($/hour)
- Tiered pricing based on live stream duration and the quality level you prefer ($/minute)
If cost is a key concern and there is a limited budget, we recommend computer-generated captions. ASR captions can usually be 10 or 20 times cheaper than human-generated captions with the trade-off being lower accuracy.
In summary, there is a growing demand for live captioning for events and meetings to increase the accessibility of content. Human captioning services offer higher accuracy than machine learning based captioning services but are typically more expensive.
To learn more about Live captioning, please visit the following posts:
References:
Ai-Media, ‘Should You Use Computer-Generated or Human-Generated Captions?’, Ai-Media, https://www.Ai-Media.tv/should-you-use-computer-generated-or-human-generated-captions, (accessed 02 October 2020)
Ai-Media, ‘External Captioning Quality Audit’, Ai-Media, https://www.Ai-Media.tv/external-captioning-quality-audit, (accessed 02 October 2020)
Allison Koo, ‘How to Calculate Word Error Rate’, Rev, https://www.rev.ai/blog/how-to-calculate-word-error-rate, (accessed 02 October 2020)
EEG Enterprises, ‘EEG iCap’, EEG Video, https://eegent.com/icap, (accessed 18 October 2020)
EEG Enterprises, ‘Lexi™ Automatic Captioning Service for Live Video’, https://eegent.com/products/QAM44XW07EVXHHZS/lexiTM-automatic-captioning, (accessed 18 October 2020)
Epiphan Systems Inc, ‘Epiphan LiveScrypt’, Epiphan Video , https://www.epiphan.com/products/livescrypt/, (accessed 05 September 2020)
Romero-Fresco P., Pérez J.M. (2015) Accuracy Rate in Live Subtitling: The NER Model. In: Piñero R.B., Cintas J.D. (eds) Audiovisual Translation in a Global Context. Palgrave Studies in Translating and Interpreting. Palgrave Macmillan, London. https://doi.org/10.1057/9781137552891_3